Common misconceptions about generative AI
Generative AI doesn’t just generate content -- it can spark diverging opinions about its value. It offers many benefits, but diligence is necessary to realize its value.
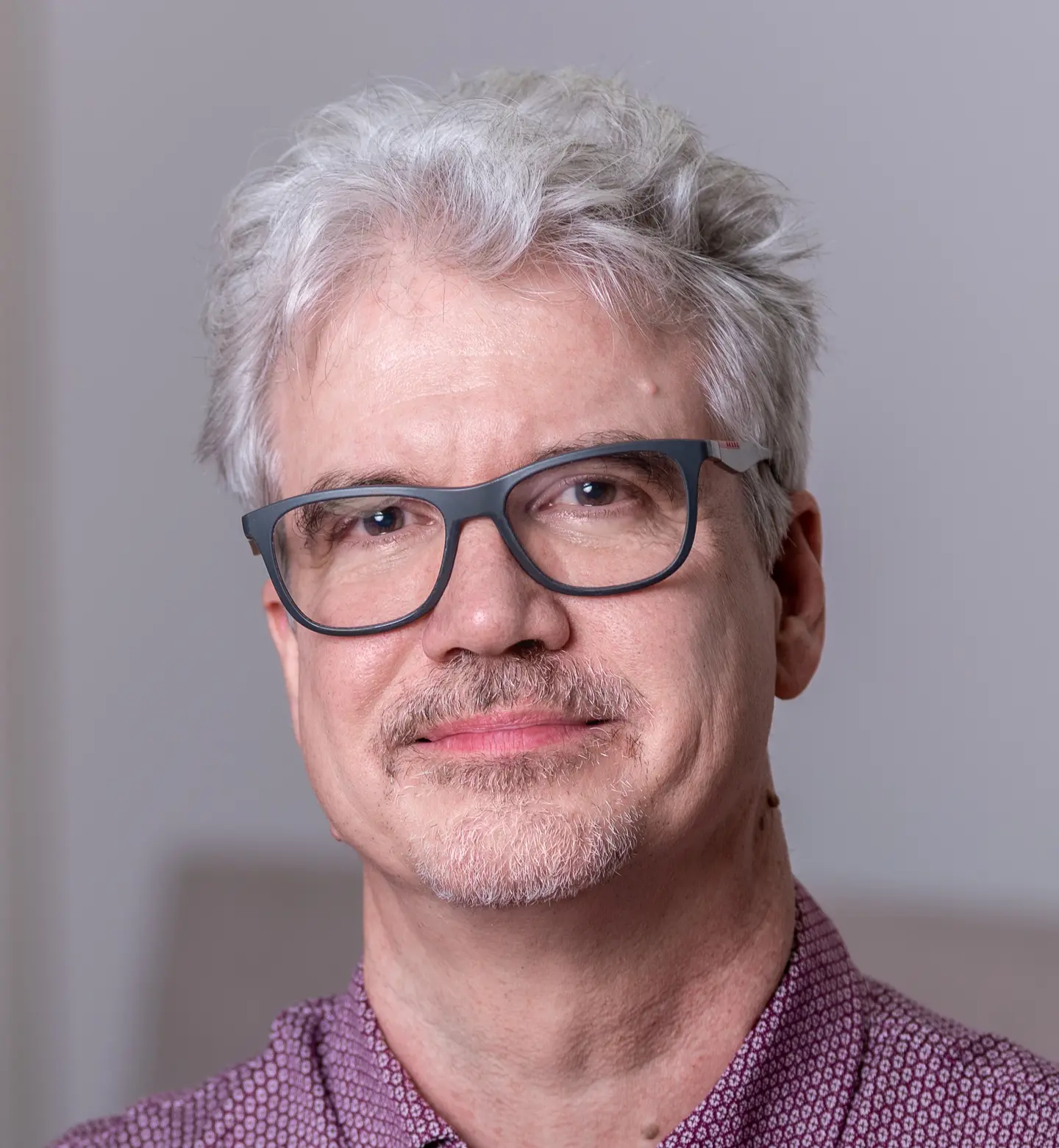
Published on Jul 13, 2023
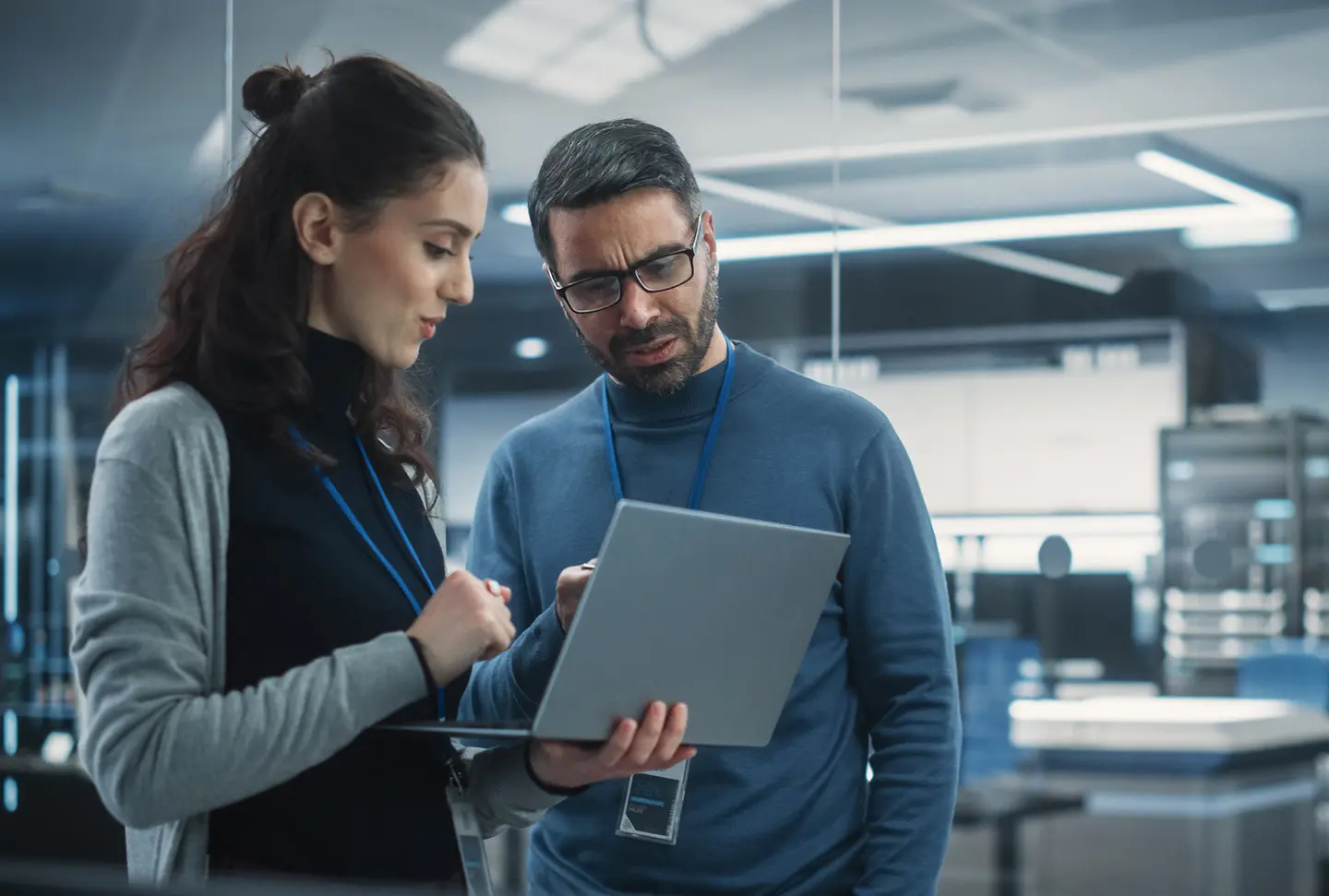
Generative AI doesn’t just generate content -- it can spark diverging opinions about its value. It offers many benefits, but diligence is necessary to realize its value.
Published on Jul 13, 2023
Generative AI is a transformative technology. Enterprises should start exploring their capabilities and not hold back to see how competitors adopt them. At the same time, firms also need to have realistic expectations about what generative AI can and can’t do.
Large language models (LLMs) that enable generative AI tools such as ChatGPT have vaulted into public awareness quickly, especially in comparison to other recent technologies. Rather than slowly building public awareness and understanding, LLMs have gained sudden fame or notoriety, depending on your perspective.
Many people now have some experience with LLMs – enough to form an opinion about them. But these impressions generally don’t provide a sufficient basis to understand the capabilities of LLMs. Common beliefs about ChatGPT and similar tools may not be entirely accurate.
Because LLMs are so new and different from familiar ways of working with content, it’s easy to dismiss them as hype or become overly optimistic about what they can offer. Many prevalent opinions reflect misconceptions, even if they contain kernels of truth.
How good is the output of generative AI, especially compared to the content people can create?
Humans are good at understanding context and nuance, while machines generally aren’t. AI bots won’t necessarily understand implicit and subtle distinctions unless they are trained to notice and respond to them. While the out-of-the-box behavior of an AI bot may seem clumsy compared to a person with expertise, the bot can be a fast learner and, in a brief time, deliver acceptable results. And when tweaked and nurtured, bots can often deliver astonishing results.
We’ve all seen the results of poorly executed AI: content that is vague, rambling, or even inaccurate. It’s tempting to fault the technology as the culprit for these problems. But while the technology does have intrinsic limitations, many times the problems are more the result of how the technology is implemented rather than the technology itself.
It can be hard to generalize about the results that are possible from AI models because their implementations are not all the same. Without knowing about the implementation, it’s difficult to know if one’s experience reflects the full capabilities of the underlying technology. Various users will get different results using ChatGPT, for example.
This misconception arises because generative AI such as ChatGPT relies on natural language inputs, which lowers the barrier to creating queries. For example, web users can now enter their own prompts into Bing and get an output. But prompt engineering is much more involved than causal prompt creation.
Good answers depend on asking the right question. In practice, prompts often require greater specificity than is used in everyday speech, which involves simple questions (What time is it?) or requests (Pass me the salt). Straightforward prompts only work if they lack ambiguity or alternative interpretations.
Most people’s experience with LLMs comes from direct-to-consumer applications such as the free version of ChatGPT studio, Bing’s chatbot search, or the numerous GPT smartphone apps one can download from an app store. These allow a single question to be asked, which is immediately responded to in one interaction – you don’t need to adjust your question or get the bot to understand something before asking your question. Zero-shot applications are good for basic questions or situations where providing general information will be adequate. But expecting someone to write a new prompt each time they need an output from AI model is not efficient.
Prompts need to anticipate potential ambiguity and alternative interpretations. While the precision of zero-shot results is improving as LLMs evolve, they often won’t provide a satisfactory answer to a more sophisticated question. It’s challenging to write a perfect prompt the first time – we often need to follow up and provide clarifications. It can be a burden for authors to constantly have to refine what they are asking for.
Developers who engineer prompts take a methodical approach, treating the prompt not just as a question but as an instruction. They specify the instruction details, contexts, input data, and output guidance. A well-engineered prompt will be one that has been tested and can be reused by many people at different times.
Many prompt instructions can be expressed in natural language, but because the prompt request will interact with a range of sources and systems, they should also include technical parameters such as weights, endpoints, or text patterns.
While non-technical end-users can create simple prompts, you’ll want to delegate the engineering of more sophisticated prompts to more technically savvy staff. They can experiment with what works and what doesn’t and then provide these optimized prompts for everyone to use.
At the other end of misconceptions about LLMs is the belief that they are all powerful and can readily take over many job roles.
This misconception is most popular with business executives who aren’t involved with writing content. Perhaps they see their school-age children using text-generation apps on their smartphones for homework and conclude that if kids can use them to write, then these bots will replace the need for dedicated writers. Some developers, always attuned to ways to save time, may also be prone to believe that generative AI can automate writing to such as degree that the involvement of writers won’t be necessary.
Those who currently develop content are less credulous about the autonomy of generative AI. Writing involves getting both the informational details correct and expressing them effectively. Before you turn content creation tasks over to generative AI, you need to make sure you can provide accuracy and audience-appropriate delivery.
ChatGPT can answer questions on almost any topic, though not necessarily with authority. Enterprises need for outputs to be accurate, specific, complete, and unambiguous. Employees and customers need to trust the output if they’re required to rely on it.
In some scenarios, generative AI can develop reliable content without active oversight. For example, generative AI could develop product descriptions based on a well-defined list of product features for each product.
But many situations involve conceptual complexity, where distinctions are important but may be hard for bots to identify or convey. Depending on how the bot is ingesting (finding and retrieving) information, it may generate text that is nonsensical, a phenomenon known as hallucination. Hallucinations occur when the data relating to the question is insufficient, inaccurate, contradictory, or lacks context.
In addition to getting the facts straight, the bot also needs to convey the information clearly.
The writing provided by off-the-shelf AI services is often not that great. Out of the box, ChatGPT delivers clunky prose that’s wordy and overly formal. The prose may not be the point, for example, rambling about the pros and cons of a topic. While AI can be trained to reflect the appropriate style, you may still need to oversee the output initially to ensure it conforms to your brand guidelines and standards.
Unless your prompts are well-tuned and you’ve determined the output is predictably reliable, you’ll want writers to interact with the bot to check the results and improve them. AI can certainly accelerate the work of content development, but it won’t necessarily replace the creative judgments or knowledge expertise that writers can offer.
Generative AI can deliver impressive results at a scale and speed that’s been previously unimaginable. But such outcomes depend on drawing on a range of inputs and services and iterating on the prompts to query those inputs. Just connecting to a generic GPT API won’t provide all that’s needed.
When engineering your own prompts, make sure your team specifies relevant resources and libraries to use and iterates and tests results. When adopting external AI services, look at what diligence the vendor has performed to ensure the results are reliable.
What if we told you there was a way to make your website a place that will always be relevant, no matter the season or the year? Two words—evergreen content. What does evergreen mean in marketing, and how do you make evergreen content? Let’s dive into it.
Lucie Simonova
How can you create a cohesive experience for customers no matter what channel they’re on or what device they’re using? The answer is going omnichannel.
Zaneta Styblova
In today’s world of content, writing like Shakespeare is not enough. The truth is, there are tons of exceptional writers out there. So what will make you stand out from the sea of articles posted every day? A proper blog post structure.
Lucie Simonova