How generative AI fits into content development processes
AI can generate content in multiple ways. Find out how to best incorporate generative AI in your content development processes.
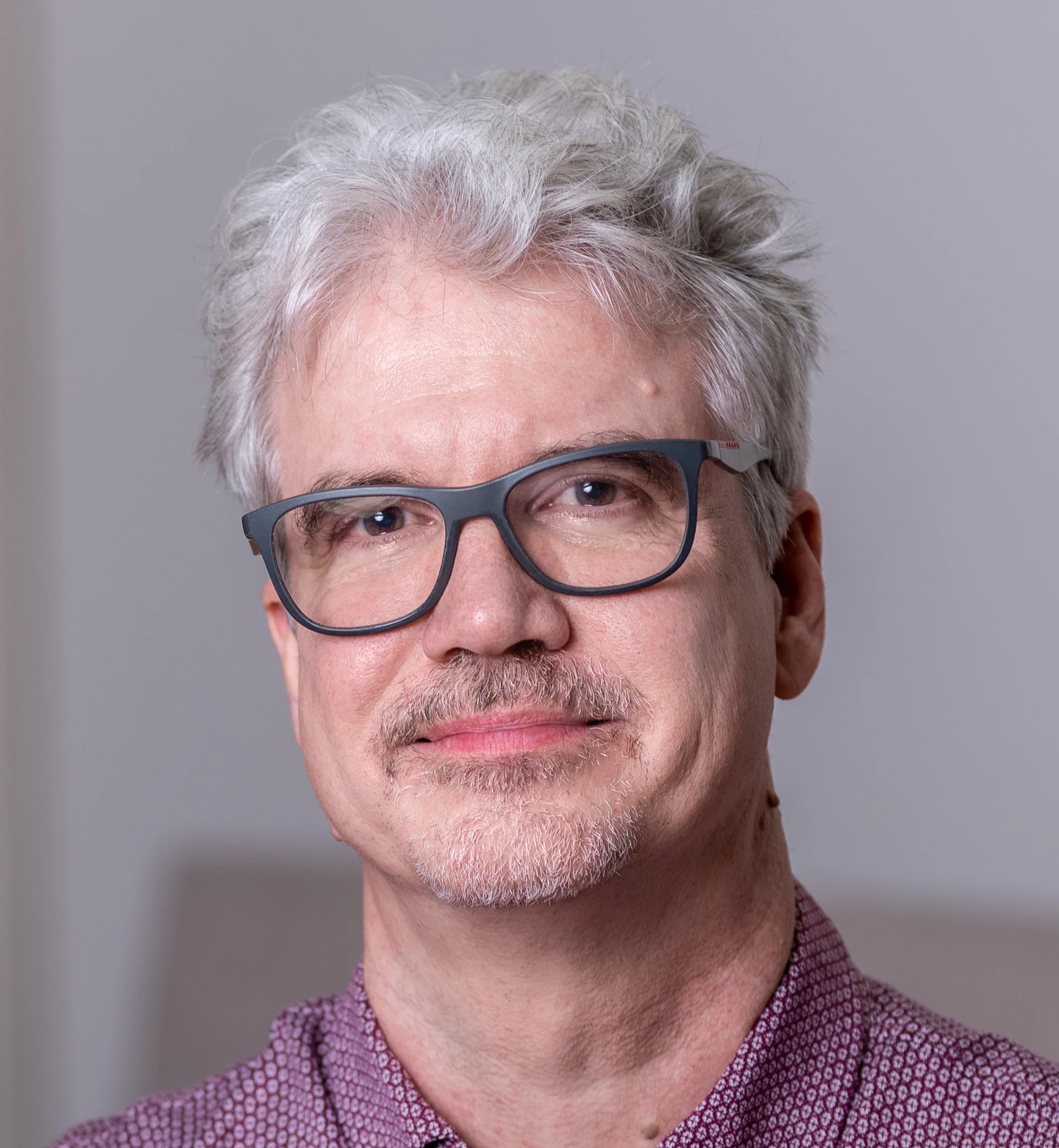
Published on Jul 31, 2023
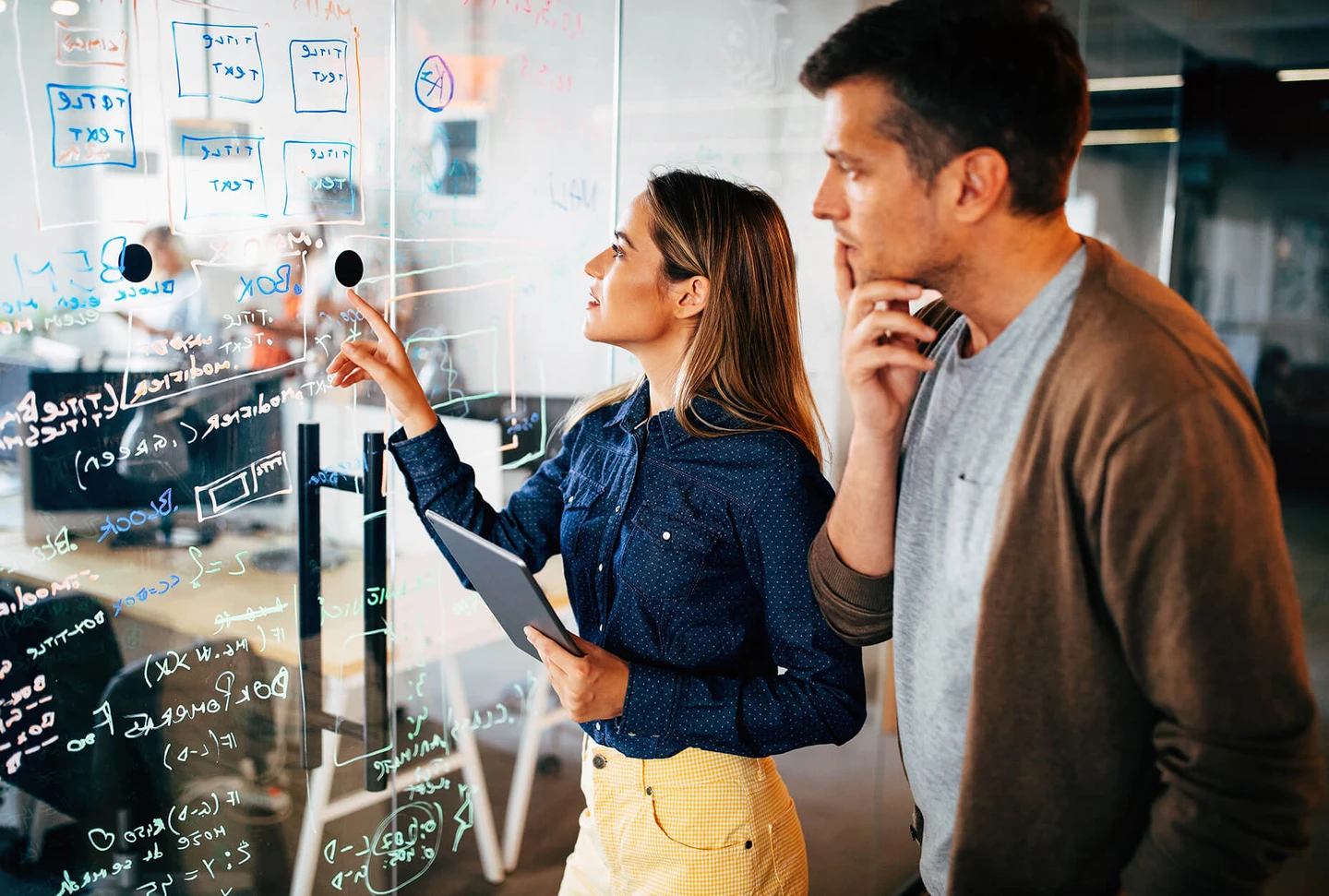
AI can generate content in multiple ways. Find out how to best incorporate generative AI in your content development processes.
Published on Jul 31, 2023
Generative AI is not another content tactic or one more product feature added to improve grammar or wording. Generative AI is far bigger than that.
Generative AI is a powerful capability that will fundamentally reshape how content is developed.
Content teams should be asking how they want to make use of generative AI. What content generation tasks can you delegate to an AI bot, and will the bot do a good job?
Content generation can be classified into two approaches: augmented generation and automated generation. We’ll look at how they differ, and why it’s helpful to be familiar with these concepts.
At Kontent.ai, we believe that AI should give you complete control over your content. You should decide the amount of control that’s right for the task. Generative AI provides many options for how it can be implemented. Choosing the right option will ensure you have control over your content.
To understand how AI can help create content, let’s consider another domain where AI is used: car navigation. Over the past decade, automakers have been pursuing how to provide a self-driving car.
Of course, no car today is truly self-driving. While full automation autonomy is the end goal – allowing us to watch a movie or take a nap while en route to our destination – the progress toward that goal is measured in incremental stages. Standards bodies and regulators classify automation into six levels, from zero (none) to five (full).
Thinking about automation as a continuum can help your team evaluate how to approach content generation. The table below shows automation levels for a self-driving car alongside corresponding levels of automation in content generation.
Automation level | Self-driving car | Content generation |
0 - no automation | Driver decides everything | Completely manual content creation |
1 - driver assistance | A single task like setting the cruise control is automated | A single feature such as automated word suggestions |
2 - partial automation | Combining tasks such as lane centering and adaptive cruise control | Combined tasks such as auto text completion and suggested rephrasing |
3 - conditional automation | Can take over driving in specific conditions, such as a traffic jam | Content generation of variations of existing content |
4 - high automation | No steering wheel, but use is restricted to certain environments | Autogenerated content based on specific, predictable data inputs |
5 - full automation | Navigation anywhere under any conditions | General purpose content generation able to answer any request appropriately |
Broadly speaking, automation is activated when a bot takes over the decision-making process. With automation, a task is entirely delegated to the bot. When people need to make decisions about bot recommendations, AI assistance augments human capabilities.
How practical is the full automation of decisions? A driverless car may be feasible in predictable environments, but autonomous navigation gets more complicated in fluid environments. Similarly, content generation becomes more challenging when the context is not routine and predictable.
While the conceptual distinctions between augmented and automated content generation may seem academic, they have practical implications. They encapsulate two distinct approaches to utilizing AI. Do you want AI to help individuals with their tasks, or do you want AI to do the tasks without people needing to be involved?
Like any dimension of content strategy, generative content poses questions about how you work with content:
The sweet spot will be use cases that deliver useful results that are practical to implement.
Your existing content development process and routines will offer clues as to whether content can be auto-generated. Content that’s routine and doesn’t require multiple people to provide feedback or quality checks will be more likely candidates for automated generation.
Ask about your current process for content development:
If the newly created content doesn’t require outside review, then automation is more likely to be feasible. Teams may decide that all content of a certain content type can be automatically generated. For example, the development of content about concrete factual topics can be straightforward to automate, provided the factual data is available and accurate. The current review may be only to check factual accuracy, making sure nothing was garbled during the rekeying or pasting of data from elsewhere. Automation would remove these quality problems, rendering human reviews unnecessary.
Success with automating content generation requires that both inputs and outputs are predictable. After implementing the prompts, teams know through testing that the output is reliable. Once it is set up, people normally don’t need to check it. It’s possible to set up a separate task that uses large language models (LLMs) to grade or evaluate the output that was generated by an LLM, providing an additional check. If an output fails the test, it can be flagged for human review on an exception basis.
Automated content generation is a prelude to autonomous content generation that would require no planning – the pre-trained large language model knows everything it needs to and can convey that information clearly, accurately, and succinctly no matter how people ask questions or make requests of it. That’s a Level 5 capability, which like the completely driverless car, is not a current reality.
When certain content routinely requires outside review, teams should document the parameters that govern the existing review process, such as what expertise is needed and what criteria are applied during the review. These parameters will be translated into rules for the bot to act on.
In most cases, people still need to be actively involved with the development of content. In AI parlance, this is known as human-in-the-loop.
Even though augmented content generation requires people to do certain tasks manually, it can nonetheless save time and increase quality compared to manual content development. A new study of over 400 professionals by MIT researchers published in the journal Science found “ChatGPT substantially raised productivity: The average time taken decreased by 40% and output quality rose by 18%.“ Generative AI tools can help all kinds of employees. Less skilled writers improved their quality dramatically, while skilled writers accelerated their output significantly.
With augmented content generation, the bot acts as a co-pilot or collaborator, making suggestions and asking questions but never dictating how the final content is created.
Augmented content generation provides greater flexibility than automated content generation, but it requires more thought about how to incorporate it into the workflow of writers, editors, and other users. Automated content generation can bypass the involvement of users. Augmented content generation, in contrast, actively engages with the user. The user experience becomes a critical factor in successful adoption. No one wants to use a pesky intrusive bot that provides poorly timed or targeted suggestions.
Users can play different roles in their collaboration with bots, acting as either the initiator or the respondent, engaging with inputs and outputs in various ways:
When presented with suggestions, users may be given a choice to accept or reject them, or else they may be given multiple options from which to choose. They may have the discretion to bypass the suggestion altogether and make their own choice.
A range of workflows can be associated with augmented content generation. These reflect different sequences for taking turns. Bots can either prepare content for people to review or they can critique or rework content the people have prepared. Humans can edit the output of bots, and bots can edit the output of humans.
Decide when the AI should be invoked in different content generation scenarios. Does the bot decide when to help, or does the user ask the bot for help?
Bots can be involved at the start, in the middle, or at the end of content development. Three workflow and interaction patterns can be used.
Generative AI is also starting to be used in video development, either in pre-production (helping to define the narrative and script) or post-production (sequence and sound editing, titling, and graphics.) In more specific use cases, generative AI is used to deliver text-to-video, where images or synthetic avatars express spoken text narratives and music.
Generative AI content development processes can involve lots of back-and-forth between the AI bot and the editor. When AI bots are involved with human-intensive tasks, the AI tool must fit into the authoring context. For high-frequency tasks especially, having native AI capabilities, rather than integrated third-party plugs, will provide a more seamless experience.
Even when people and AI bots can do the same kinds of tasks, they will do them with different proficiencies. These differences will influence whether bots take a leading or a supporting role.
In some cases, the talent or expertise of individuals will always result in better outputs than a bot on its own, while in other cases, bots will have a clear advantage over people due to the scale of their training.
For many tasks, bots are superior only in some respects. By certain measures, bots may be better at some tasks, while humans are better in other ways at those same tasks. Even where such generalizations can be made, wide performance variations within bots and individuals can operate, some of which stem from the context (human performance degrades when rushed, for example.)
People are often better suited for small-scale tasks since they can swiftly apply their judgments to develop the content that matches what’s needed. But when tasks involve scale, bots offer advantages.
Think about people and bots in terms of how they fit your priorities for your content. What is the content trying to accomplish? What constraints exist on how you can develop that content, such as the resources or time available and the scope or scale of the output required?
Consider the strengths of people and bots in terms of different priorities:
Humans are more precise with wording. When precise and nuanced wording is essential – especially the kind of wording that requires the input of multiple stakeholders – then people need to lead the content development. Bots can help with intermediate steps, such as wording suggestions, but they won’t be able to develop precise wording reliably.
Implementation context matters. In some dimensions, such as originality and accuracy, the relative strengths of people and bots are highly dependent on the implementation context. When given a directed but open-ended prompt and a large diverse LLM, bots can generate unexpected but relevant connections in their output. LLMs can be trained on a broad range of materials that can provide a rich basis for fresh ideas. But formulaic prompts, especially targeting widely discussed topics, will often result in the regurgitation of existing content rather than truly original ideas that a talented individual might offer.
The vastness of LLM training data also can yield differences in accuracy outcomes. LLMs may overgeneralize and make inaccurate assertions that overlook critical relevant details that would be known to an expert on the subject. But it is also possible for LLMs to be able to synthesize details that are present in different resources and be able to provide a more complete and accurate output response to a question than would be possible for a typical person to provide.
Where bots consistently outperform people are tasks involving scale. Because of their speed and ability to process in parallel, bots are far better at generating large quantities of content, especially when the content-to-create is based on a common prompt pattern. Bots are also efficient at creating variations of existing content.
Generative AI leverages the performance advantages of computing while reducing the technical overhead associated with software development, resulting in efficiencies for all roles involved with the content development process.
When you decide to use generative AI, should you adopt an augmented approach or move toward an automated one? Some teams may decide to start by using augmented content generation, but their end goal is automation. Other teams, given the kinds of content they are responsible for, may not be interested in ever implementing fully automated content generation. The decisions will reflect the business goals and requirements associated with specific content types and topics.
Automation necessitates greater upfront effort to engineer prompts through iteration, though it yields greater savings of time after setup than an augmented approach to content generation.
Plan for surprises. A key consideration is how much the bot will need to adapt to different circumstances. Two factors play a prominent role in how well bots can adapt:
The more that bots understand the immediate context, the more adaptive they will be.
Start simple with your prompts, then evaluate what’s missing and add or eliminate parameters that yield outputs that more accurately reflect intentions.
This article has looked at some strategic considerations when deciding when and how to implement generative AI in your team’s content development process.
The right decision will depend on the use case. For example, how much can generative AI help with a task such as writing a blog post like this one? Blog posts are typically unique content, which will reflect a range of requirements, such as the audience, their interests, and questions or interests to address. At their best, blog posts convey something that will be new to the reader. While it’s possible to have a bot write blog posts, that tactic is not likely to result in content that will be unique in its information or perspective, meaning that the output will risk saying the same material that has been conveyed in other content the reader may have seen.
After I started writing this post, I asked ChatGPT a simple question about the difference between augmented and automated content generation. The answer, while entirely accurate, wasn’t that interesting. A behavioral trait of ChatGPT is its tendency to answer broad questions with sweeping generalities. The answer stated things I already knew. Admittedly, I know more about this topic than many folks, but I still was hoping for some fresh insights. I set the response aside for a few days, and when I read it later, I noticed in the output a minor general comment relating to use cases for augmented generative AI, which I began thinking about. The response didn’t articulate the idea very robustly or elaborate on it. But it offered the kernel of an idea that I hadn’t thought about previously and which I decided needed more investigation and development.
AI can speed up many content development tasks. But sometimes, to get the most value out of it, you also need to slow down enough to identify where it will add more value. The most innovative results from content generation arise through the iterative exploration of questions and outputs.
What if we told you there was a way to make your website a place that will always be relevant, no matter the season or the year? Two words—evergreen content. What does evergreen mean in marketing, and how do you make evergreen content? Let’s dive into it.
Lucie Simonova
How can you create a cohesive experience for customers no matter what channel they’re on or what device they’re using? The answer is going omnichannel.
Zaneta Styblova
In today’s world of content, writing like Shakespeare is not enough. The truth is, there are tons of exceptional writers out there. So what will make you stand out from the sea of articles posted every day? A proper blog post structure.
Lucie Simonova