AI-based auto-tagging of content: What you need to know
Like other AI technologies, auto-tagging is generating growing interest. Is auto-tagging right for your organization’s content? Find out what you need to know about this emerging capability.
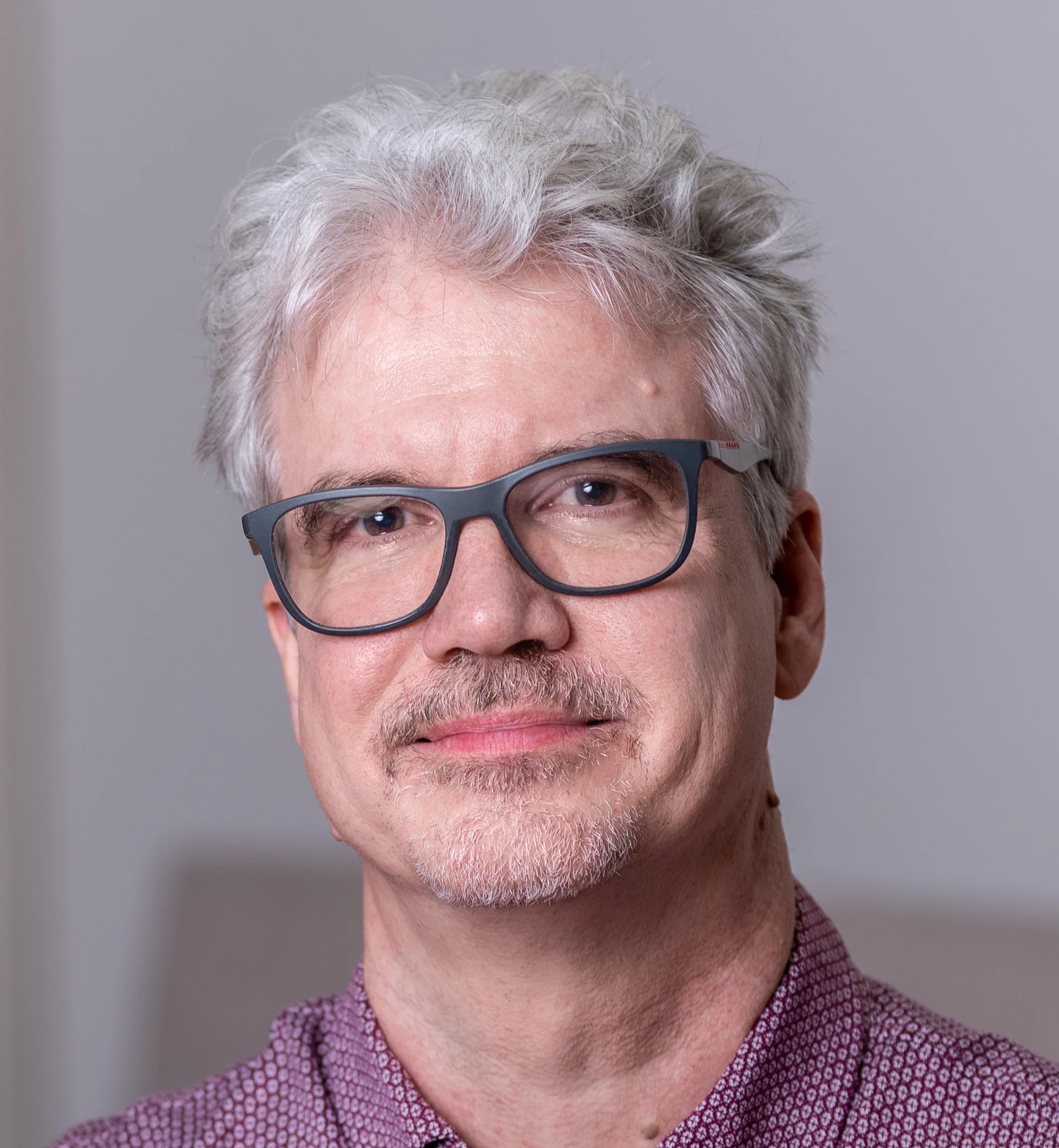
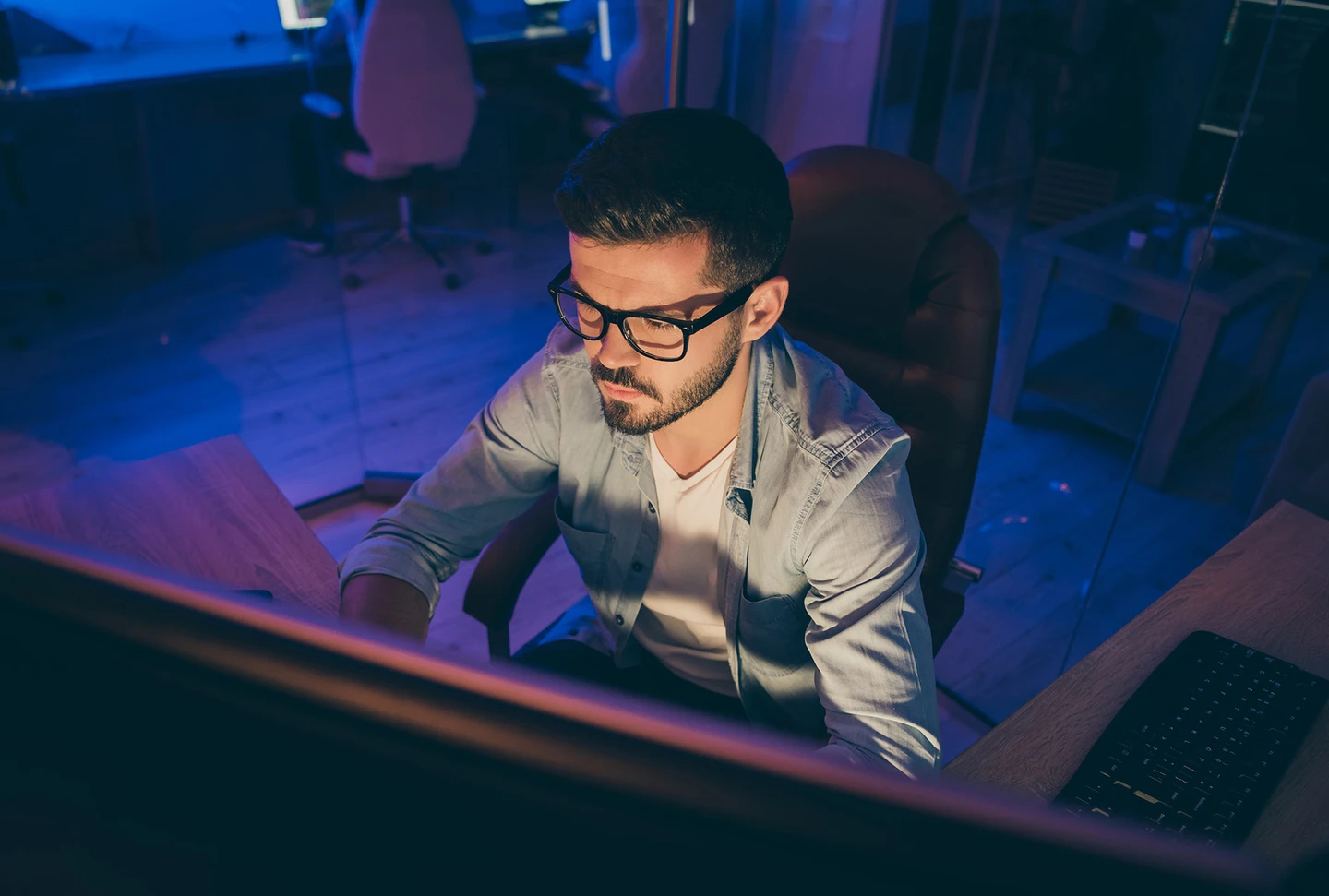
Like other AI technologies, auto-tagging is generating growing interest. Is auto-tagging right for your organization’s content? Find out what you need to know about this emerging capability.
Tags help people and machines understand what content items are about. They are important because they help to locate the right content that’s needed to support any stage of the content’s lifecycle, whether it’s revising existing content, integrating pieces into large content items, delivering personalized content, or assessing content performance.
Tags offer a standard language that can be used across the organization to manage and coordinate its content. Providing the right content by relying on tags depends on getting the tags right. That’s where interest in auto-tagging comes from. It promises a better way to add tags to content.
Tags are about terminology, so let’s clarify the terms we will be using. Tags are a kind of content metadata (but be aware that other kinds of metadata exist that aren’t tags). Tags identify what the content is about according to a defined list of taxonomy terms, which are standardized descriptive keywords. Because content tags use standardized terms, they are different from social media hashtags, which are normally user-defined.
Tagging is a manual or automated process by which the content is described and labeled with taxonomy terms.
Auto-tagging refers to the automated application of tags to describe content. Note that auto-tagging for description is different from the campaign tags used by Google for ad tracking purposes, which Google also refers to as auto-tags.
Developers have explored ways to reduce the manual effort to tag content to speed up the process and increase its application. Some common approaches include:
This discussion will focus on AI-based solutions to add tags to content, which is more sophisticated than bulk or rule-based approaches. AI-based software can offer the greatest flexibility and can run autonomously once set up. These approaches are also evolving quickly.
Auto-tagging can be used with both text and images, though the process used for images will be different than for text.
Identify sources of text content. The text you publish may come from either internal or external sources.
Authors in your organization will create content, and as part of the workflow, metadata tags will be added. Software can automatically add tags by evaluating the text, which streamlines the content development process.
Another possibility is user-generated text, such as reviews and feedback from customers. Some e-commerce vendors, for example, add tags to product reviews to note specific comments made relating to durability, convenience, or satisfaction. Such text won’t have a defined workflow, meaning that automated tagging is especially beneficial. Auto-tagging will add labels nearly instantly that customers can use to sort through reviews addressing features of most interest to them.
Images, especially photos, can benefit from auto-tagging. Without tags, images can be hard to search and locate. Images of similar items can sometimes be difficult to distinguish easily, meaning that people may access the wrong image. Auto-tagging can identify what is in an image, such as people, locations, settings, objects, and colors. Many retailers may use auto-tagging to tag visible product features.
Auto-tagging of audio and video is also possible but is generally more limited in its application. AI software is starting to recognize segments and events, but most automated classification relies on text descriptions and titles rather than the contents of the audio or video source file.
For a long time, publishers have had trouble tracking and managing the vast quantities of content they produce. They’ve needed easier and more reliable ways to add tags to their content. The New York Times describes the appeal of automation: “Extracting structured data from text is a common problem at The Times, and for 164 years the vast majority of this data wrangling (e.g., cataloging, tagging, associating) has been done manually. But there is an ever-increasing appetite from developers and designers for finely structured data to power our digital products and at some point, we will need to develop algorithmic solutions to help with these tasks.”
The use of AI has simplified the tagging of content items. What was once a time and attention-intensive task now promises to become much easier. The software can do the heavy lifting:
Provided the software performs accurately, the burden on authors and other people supporting content operations is greatly reduced.
Before embracing any auto-tagging solution, it’s important to understand how it works, its strengths and weaknesses, and the up-front investment required.
Auto-tagging software looks for a match against a linguistic, logical, or mathematical profile it is expecting and recognizes. It can rely on a range of technologies:
Some auto-tagging software will rely on a specific technology. For example, image tagging will normally utilize machine learning. Lots of auto-tagging software requires “training” of some kind by showing examples of correctly tagged content and then feeding untagged content to see if it gets tagged correctly. If the software can’t determine the correct tag, humans will provide the correct tag by inserting missing tags and by removing or changing wrong tags.
The tagging of text can use various kinds of technology and will sometime use several technologies in combination. The New York Times reviews the text in cooking recipes to extract data from them by making probabilistic guesses. While auto-tagging can achieve a reliable level of accuracy, it is rarely completely accurate. The degree of accuracy achieved and the level of effort required to correct inaccuracies will be factors to consider when evaluating the utility of an auto-tagging solution.
Is auto-tagging good at applying taxonomy labels to content items? It can be. But auto-tagging also has limitations.
Auto-tagging is not suitable for applying certain kinds of taxonomy terms. Remember, auto-tagging will only be able to assess what is within the content, such as entities that are present. If the publisher needs to label the content with a description of the intent of the content, which is not explicit in the text or image, it can’t rely on auto-tagging. Such external information may relate to the intended audience for the content or the stage of the user journey. These tags will still need to be added manually.
Auto-tagging can be limited by the characteristics of the source content. Your success will depend on whether you are able to describe the specific information that users want. Sometimes there’s a gap between what auto-tagging produces and what users need. The descriptions might be too vague, or they miss critical elements.
The accuracy of auto-tagging is measured by two dimensions: precision and recall. In a study on the auto-tagging of marketing content published in the Journal of Business Research, a global team of researchers explained these concepts: “Precision measures how well the model avoids assigning the wrong keyword to an article; it is the number of true positives, positive instances that were classified correctly, divided by the sum of true positives and false positives (negative instances that were classified as positive). In contrast, Recall measures how well the model assigns keywords correctly to an article; it is the number of true positives divided by the sum of true positives and false negatives.”
In short, you want both high precision (avoiding tagging with the wrong terms) as well as high recall (avoiding missing correct tags).
Auto-tagging software can make incorrect classifications, or it can overlook classifications it should have made.
Inaccurate tagging is not always immediately apparent. But in some cases, tagging errors can be blindingly obvious, such as when a photo of a breakfast pastry is classified as a dog.
The quality of auto-tagging output will depend on whether it has reliable examples to train upon and whether future content to tag will resemble prior content. Quality will be influenced by:
Auto-tagging shines in certain situations but struggles in others.
When it works well, auto-tagging brings greater efficiency and consistency to the tagging process. It can bypass some of the friction associated with manual tagging, such as poor staff understanding or commitment to tagging, the uneven application of tagging, and errors or inconsistencies in the tags used. Manually tagged content, if done poorly, can cause systems that depend on the tags to become unreliable.
Keep in mind that no tagging approach will be 100% accurate all the time. One question to consider is whether human errors or machine errors will generate more problems for tag-dependent use cases.
Auto-tagging provides strong benefits when:
Auto-tagging can be an effective solution, for example, when needing to migrate a large volume of content items that are well understood.
Manual tagging is a better option when human judgment is necessary.
The table below summarizes some key strengths and weaknesses of each approach.
Strengths | Weaknesses | |
Auto-tagging | More consistent | Only works in well-understood domains |
Manual tagging | Better in novel situations | Prone to unevenness |
While the ideal of automation is that you can press a button and instantly have the work done for you, the reality is not that simple. Before you can have any process run autonomously, you’ll need to first invest in some preliminaries:
Envision what you want. Organizational planning concerns how your enterprise expects to utilize auto-tagging. First, you need to define what success looks like. Define clear goals for auto-tagging, including:
Map out your process. Once you decide what you aim to achieve, you’ll want to decide how you would like to accomplish that. You’ll want to design a process for how auto-tagging will happen. The process will address workflow and procedures such as:
Get your inputs ready. Your readiness will depend on whether your content and taxonomy terms are sufficiently complete to allow AI to do the auto-tagging.
The detail of the content needs to match the granularity of the taxonomy describing it. For example, attempting to auto-tag content based on the title of the content alone would probably be insufficient. Likewise, a shallow taxonomy hierarchy may have difficulty connecting to content that addresses very specific details.
Once your content and taxonomy are ready, it’s time to validate whether your taxonomy terms can be matched to your existing content.
The setup of auto-tagging software is not currently a simple plug-and-play operation. You’ll need to train your tool to understand your content.
Auto-tagging tools not only employ diverse technologies but also focus on different problems. Some are agnostic about the nature of the content they evaluate, while other tools have been designed for a specific kind of content.
One size doesn’t fit all needs. You may decide to use more than one tagging tool, depending on the kinds of content you want to tag and the goals for your tagging. For example, you may use one tool to tag articles and a different one to tag photos.
Broadly speaking, auto-tagging tools can be grouped into three categories:
Classification services apply the power of big data to the classification of your content. These AI services have been trained on vast repositories of content, which may – or may not – resemble the content your organization develops and publishes. Services such as Amazon Rekognition are available via an API. They offer generic classification capabilities that are not domain specific.
Metadata management solutions increasingly offer auto-tagging options. Metadata tools such as Poolparty manage enterprise taxonomies and have APIs that can connect to content repositories to auto-tag the content. Most metadata tools focus on auto-tagging text content and will often rely on semantic classification approaches. The quality of the auto-tagging will be dependent on the semantic model managed by the tool.
Vertical solutions have emerged recently to address specific domains, especially e-commerce. These tools are specialized and will be dedicated to performing a narrowly defined task, such as tagging photos of fashion garments to identify their visual features and characteristics. Vertical solutions sometimes have simpler setups, but they will often be more restricted in the range of tagging they can perform.
Be sure to evaluate whether a tool does what you need it to. Some tools may offer capabilities that your organization won’t need or will require more effort than you’re prepared to make. Cross-check promises made in vendor demos by doing a proof-of-concept evaluation.
The implementation of auto-tagging has grown in recent years, though the approach is still in its early stages of adoption. Tapping its benefits requires an up-front investment.
Success requires learning how to work with the technology in your specific context. The best way to determine your auto-tagging implementation strategy is to separate procedural issues relating to tagging your content with taxonomy terms from the technical issues relating to the software itself.
Debug your manual tagging process. Don’t jump straight into auto-tagging. First focus on refining your manual tagging process, so that you don’t end up automating problems. Make sure you understand the characteristics of your content and your taxonomy. Clarify roles and responsibilities. Close any gaps in your taxonomy.
Once you are confident that your manual tagging process works, you can begin to automate it.
Train your software. You’ll generally need to have some content that’s already been tagged correctly to train the software. Researchers writing in the Journal of Business Research note that it is best to start the software training process with content that’s been tagged by the content creators manually, which provides a “ground truth” (i.e., known taxonomy terms to evaluate the model against).
Pilot and check. Once you get the software trained to produce reliable tagging, you are ready to implement it on a pilot basis for content your organization publishes. But technical viability does not mean that the implementation will be ready to work fully autonomously. Until you have enough experience to know that the implementation is reliable, it’s recommended to set up a “human in the loop” (HITL) process where people will:
Over time, the amount of human intervention can be reduced until it may not be needed. But in cases where accuracy is paramount, you may decide to always include human review even if your process is generally reliable.
What if we told you there was a way to make your website a place that will always be relevant, no matter the season or the year? Two words—evergreen content. What does evergreen mean in marketing, and how do you make evergreen content? Let’s dive into it.
Lucie Simonova
How can you create a cohesive experience for customers no matter what channel they’re on or what device they’re using? The answer is going omnichannel.
Zaneta Styblova
In today’s world of content, writing like Shakespeare is not enough. The truth is, there are tons of exceptional writers out there. So what will make you stand out from the sea of articles posted every day? A proper blog post structure.
Lucie Simonova